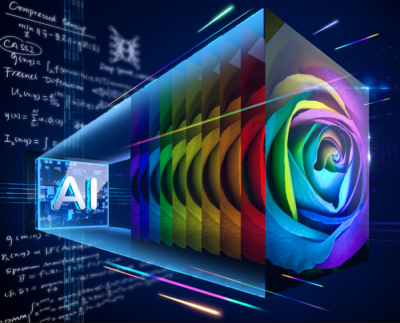
Spectral images are widely applied in remote sensing, medical detection, food inspection and other fields. Traditional spectral imaging techniques obtain spectral images through scanning, which has a long acquisition time and large system volume. As computational optics develops, researchers tend to make some encodings in the spectral image acquisition process and resort to iterative optimisation algorithms for spectral reconstruction. However, such methods also have many burdens on computation, and usually need minutes or even hours for one reconstruction. In recent years, deep learning has shown its power for science and application, and spectral imaging is not an exception. When spectral imaging equips with deep learning, it can make reconstruction within seconds, together with high spectral resolution and compact systems.
A team of scientists, led by Professor Xiang Hao from Zhejiang University, China has reviewed recent developments in deep-learning-empowered spectral imaging. They gave an overview of how deep learning is applied in spectral imaging, as well as fundamental principles and comparisons of state-of-the-art techniques. They also arranged today’s available spectral datasets for the benefit of researchers in the field of spectral imaging and deep learning.
Making effective categorisations is essential in writing a review article. The authors believe that they have found the desired categorisation approach for deep-learning-based spectral imaging. “We group various learned spectral imaging methods into three categories—amplitude-coded, phase-coded and wavelength-coded—based on the basic characteristics of light.”