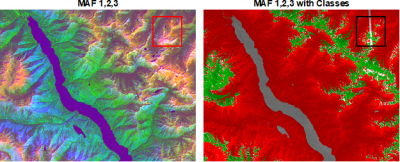
A new Open Access paper in JSI—Journal of Spectral Imaging compares common factor-based methods for hyperspectral image exploration: principal components analysis (PCA), maximum autocorrelation factors (MAF), minimum noise factors (MNF) and maximum difference factors (MDF). These can all be posed as a maximisation problem that ultimately yields a symmetric eigenvalue problem (SEP) for each model.
The SEPs allow a simple comparison of the models for analysis of hyperspectral images using a PCA metaphor with MAF, MNF and MDF describable as weighted PCA. The examples in the paper showed how the different methods captured different signals in the images and the scores from each method can be combined synergistically allowing for additional modelling and extended visualisation. MDF is a factor-based edge detection model that not only allows for additional visualisation but the opportunity to identify and exclude (or highlight) edge signal in the images. The third example showed that models can also be used synergistically for finding and elucidating anomalies. In the example, MDF showed the highest sensitivity of the models studied for anomaly detection.