Stéphane Brochot
CASPEO, Orléans, France. www.caspeo.net
Editor’s summary
As defined in the “Code of Practice for Metal Accounting”,1 Metal Accounting is part of Financial Accounting and helps in defining production costs and revenues, as well as stocks and WIP (Work In Progress) inventories. It is also the baseline for estimating the net value of the company. Metal Accounting is based on reconciled material balance which is itself based on critical measurements. Any uncertainty in measurement, due to the inevitable measurement error, in which the sampling error is generally the main component, results in an unwanted—and unnecessary—financial risk. Two examples are presented below together with associated economical risks and losses.
Example 1: Underestimating losses and overestimating metal in WIP
A custom copper smelter processes concentrates coming from numerous mines around the world. After blending, the concentrates are processed through a flash smelting furnace producing copper matte. The main copper losses at this stage are through slags and fumes. The latter are made up of fine particles containing copper, which are recovered and recycled to the furnace. But slags constitute a significant real loss of copper.
In this example, granulated slags were manually sampled on the conveyor belt discharge with one increment every two hours, collectively constituting a daily composite sample. To perform a quality control variogram analysis, a specific sampling campaign was performed by taking one increment every 15 min and analysing each increment. Though most increments had a copper content close to the usually observed daily average content for slags, several showed a significantly higher content corresponding to spots of matte entrained by slags. With the old sampling approach, such spots are “hidden”—hidden from view and hidden from metal accounting. For the baseline purpose it was then decided to install an automatic cross-stream sampler at the discharge end of the belt conveyor, taking one increment every 15 min.
For comparison, over one month, the old sampling method continued to be performed in parallel with the new, much more frequent approach. The average content for one month was 0.66 % with the old method and 0.95 % with the new automatic sampler, which is a significantly large difference when accumulating over time. It is clearly a bias as the day-by-day analysis indicated that the copper content was slightly lower for 5 days with the new sampler, but significantly higher for 13 days.
Considering a production of 1000 t/day of slag during 350 days in the year, and a price of US$9400 per ton of Cu, the value of the revealed copper loss can easily be calculated:
Loss = 350 × 1000 × (0.95 % – 0.66 %) × 9400 = US$9.541 million
This sampling issue has two financial impacts:
- Significantly, one part of copper is reported with slags due to thermodynamic equilibrium between slag and matte phases. But another part is due to entrainment of matte with slags in the form of matte droplet. This is what occurs when observing “spots” of high copper content. This is due to poor control during the slag and matte discharge process. By observing this effect and the operating conditions when it appears, it is possible to improve the process control strategy, specifically for the quantity and quality of the furnace feed, provided the feed control, based on sampling, is sufficiently accurate to avoid such spots. The recovered monetary value will be able to pay for installing an accurate cross-stream automatic sampler, which will provide a much-improved regular analysis enhancing the possibilities for furnace control. It can also pay for a well-designed automatic sampler for feed control, or, much better, an online full-stream analyser that will drastically reduce total process sampling errors.
- When establishing the material balance for metal accounting, such hidden losses run the risk of impacting also on the intermediate stocks (WIP) estimates. Indeed, some of these, such as matte skulls, dust or converter slags, are very difficult to measure in mass, but more seriously also for copper content. Consequently, typically no measurements are performed, or if any are carried out, they will unavoidably result in large unwanted measurement uncertainties. When running data reconciliation, the imbalance due to biased slag sampling is counterbalanced by the less accurate parameters associated with the relevant WIP, namely their Cu content. Month after month, the overestimate of Cu mass accumulates as an overestimate of the material mass and of its Cu content. At the extreme limit, Cu content can exceed 100 % (physically absolutely impossible of course) or inventories may report large WIP masses, which de facto do not exist. When such discrepancies between accounted inventory and reality are revealed, an accounting adjustment will have to be made, which will decrease the value of the company and, ultimately, give it a bad reputation on the stock market—many negative cascade effects can arise from neglect of basic sampling quality requirements.
This example shows how a poor sampling procedure can hide a potential route of revenue improvement and can generate a financial risk at the level of several millions of US$ per year.
Example 2: Copper concentrate trading
A custom smelter buys copper concentrates following this procedure:
- The copper concentrate delivery is accepted based on “provisional data” provided by the seller: the wet mass of the material, the average moisture content, the inferred dry mass and the average metal contents.
- During the concentrate delivery unloading, the mass of the wet material is measured by the buyer and samples are taken for determination of moisture and metal contents, constituting the “smelter data”.
- Finally, after a few months, negotiations between seller and buyer ends with a set of mutually acceptable “final data” which are then used to calculate the objective value of the delivery for final invoicing. If too large discrepancies are observed between provisional data and smelter data, an umpire laboratory can be used to redo sample analysis and a conformity assessment organisation will be asked to control mass measurement systems as well.
Provisional data and smelter data come from “measurements” which are inherently uncertain for mass, moisture content and Cu content. A representative of the seller can be present during delivery unloading to validate the wet mass measurement and the moisture content determination. In that case, the final value for dry mass is defined during the delivery, and the negotiations are focused on metal content only.
The delivery unloading is carried out using a belt conveyor. A static belt weigher measures the mass per batch (batches are typically scaling at approximately 5 tons). The following cross-belt automatic sampler is taking one primary increment per this batch mass (5 t). 100 increments corresponding to a lot of 500 tons are combined for copper analysis.
This standard procedure gives a relatively good precision. Typical relative measurement errors are 0.21 % for the wet mass and 4.9 % for the moisture content, giving a 0.5 % error for the dry mass and 0.66 % for the Cu content. The last value corresponds to TOS-correct sampling, but it is well-known that cross-belt samplers (also named hammer samplers) cannot provide correct, bias-free sampling.
Considering, for example, a delivery of 17,000 tons with a provisional Cu content of 26.000 % and a smelter Cu content of 25.825 %, this analytical difference is acceptable by both parties, because it is of the same magnitude as the measurement error for Cu content. This difference corresponds approximately to a value of US$280,000 (estimated for copper alone). But if the smelter uses a poor-quality sampling system, its results will not be able to influence the negotiation so that the final value will be closer to the provisional value provided by the seller, which has assuredly slightly overestimated—this is not good for the smelter.
Conversely, a high-quality sampling system, associated with an efficient metal accounting system (data reconciliation reducing the uncertainty in the delivery quality estimate), will tip the scales in favour of the smelter. This positive economic difference can represent up to about US$1 million per year for such a wise smelter.
Conclusions
These two examples demonstrate with great economic clarity the advantage of adapting accurate (bias-free) sampling systems, based on the Theory of Sampling (TOS), as verified by competent persons,1 to limit the financial risk from hidden evidentiary lacunae—and generate revenue instead. Only copper has been considered here, but other sources of revenue such as precious metals—or penalties associated with undesirable components—will also be impacted by the quality of the sampling system. This is why any investment in accurate and efficient measurement systems, including sampling systems, will very often be counter-balanced by the associated revenue increase. This will also be able to pay the costs of system maintenance, which is vital to maintain the stringent level of accuracy needed for proper metal accounting.
Reference
- AMIRA, P754: Metal Accounting – Code of Practice and Guidelines. Release 3, February 2007.
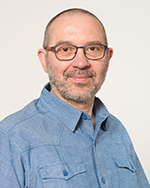
Stéphane Brochot
Stéphane Brochot obtained his doctorate (PhD) in physics from the University of Orleans-Tours and completed his education with a degree in mathematical engineering and computer processing. He joined BRGM (the French Geologic Survey) in 1991 as a researcher and as head of software development. Since 2004, Dr Brochot has been co-managing director of Caspeo, where he continues research on mineral processing modelling and simulation, sampling, data reconciliation and metal accounting. Brochot is one of the inventors of INVENTEO. His extensive expertise in sampling has been used for several mining companies and other industries requiring sampling of solids.