Quentin Dehaine
Senior Researcher at the Geological Survey of Finland (GTK), Circular Raw Material Hub (Vuorimiehentie 2) 02151, Espoo, Finland
Grab sampling for material accounting
A small polymetallic mining operation intended to evaluate the potential for upgrading its mineral processing plant by adding a new metal recovery line at some strategic point in the circuit. The objective was to recover some of the companion metals as by-products of the main commodity. These potential by-products had so far been disregarded because of non-favourable market prices, relatively low grades and lack of ore-body knowledge. A geometallurgical assessment revealed that these metals were hosted in suitable mineral phases and could be easily recovered though further concentration. The circuit itself was relatively simple with very little recirculation, each stream of the circuit branching out from the main route being sent for stockpiling or disposal in waste piles. To evaluate at which location in the circuit upgrading would be optimal and which strategies to implement, a thorough material balancing was needed in order to assess the overall distribution of the metals in the different plant streams, and in particular in the so-called residues, in terms of metal grades and recoveries.
The data available for this case is a combination of on-line sensor data (flowmeters, belt weighers etc.) as well as analytical assays from samples collected at various locations in the circuit, or from some residue/wastes stockpiles; the data covers one month of operations.
A first attempt at reconciling the comprehensive data base showed huge variations between the initial and reconciliated data for the main commodity (± 50 %) and significant discrepancies between metal accounting and real production outputs, which were even larger for the lower grade by-product metals (deviations up to ± 150 %). This issue was not new and, as a consequence, an external audit was conducted in order to evaluate the sampling procedures in use.
Three main points of concerns were raised:
- The coarsest residue streams were sampled by grab sampling “all around the perimeter” at the bottom of the stockpiles only.
- Sampling of a hydrocyclone bank overflow. Indeed, the operator instead of collecting the whole overflow stream from the collecting tank, was systematically collecting the overflow of one hydrocyclone only (the most accessible one).
- The primary pulp samples were stored in “big bags”, which were not leak-proof and which were in fact used to drain out the water “without losing the sample”.
All of these sampling practices are in opposition to the TOS’ basic principles, whereby a sample is considered representative only if all particles making up the lot have the same probability to end up in the final sample. The sampling practices revealed by the audit were clearly not in compliance with this cardinal rule and were identified as responsible for the metal accounting discrepancies observed.
The technical explanation as to why, and how to remediate these deficiencies follows.
- Grab sampling is a very dangerous practice as it generates a range of sampling errors (GSE, IDE and IEE), most of which cannot be quantified, nor corrected for. Even though this has been known for a long time at the mine site, grab sampling was still thought to be “good enough” by the operator and by management. In the present case, the grab samples collected from the stockpiles typically weighed around 20–30 kg, too small to be considered representative! Indeed, getting a representative sample (i.e. TSE < 20 %) from the corresponding stockpile, some of the coarsest streams would require at least a 20–30 ton sample. Remedial action: Sampling the same materials, but at the discharge point of the corresponding conveyor belt feeding the exact same stockpile, using composite sampling, would achieve a TSE of about 4 % with only a 20 × 20 kg aggregated sample. This will result in a representativity which is 20 / 4 = 5 times improved, for one or two hundredth of the weight (i.e. 400 kg as opposed to 20–30 tons). Not included yet in this balance is the huge time and efforts saved, which, of course, will also impact in the bottom line significantly.
- By only extracting from the most accessible hydrocyclone overflow stream from a bank of six hydrocyclones, the operator was effectively only sampling one-sixth of the whole stream and, therefore, committing several serious sampling errors (GSE, IDE and IEE). Worst, these errors were systematic as the operator always sampled the same hydrocyclone. Remedial action: This is, of course, an issue that can easily be overcome by sampling the whole output stream—especially as the output hydrocyclone overflow collection tank is located only a few metres away from the position of the present calamity.
- In metallurgical accounting, the moisture content and wet mass of the material being sampled is of equal importance as metal grades for determining the mass of contained metal in a given stream.1,2 By allowing pulp samples to “dewater” from non-leakproof big bags before measuring the moisture content, the operator introduced an enormous bias in moisture content estimation and, therefore, also in the estimated metal content after reconciliation. Also, this procedure resulted in a considerable loss of ultrafine particles (slimes), which “happen” to contain significant amounts of some of the desired by-product metals, but were lost with the uncontrollably leaking water. Remedial action: This issue was easily overcome by placing the pulp samples in impervious sealed containers for immediate delivery to the weighing and moisture determination station.
The TOS’ universally optimised 1D sampling approach
Large heaps, stockpiles or similar storage facilities cannot be sampled in situ (grab sampling). They can only be sampled correctly (in theory and practice) through a lot of work, such as transfer or displacement of the whole lot, which is often not practical and always costly. Indeed, such (very) large multi-modal lots are frequently also extremely heterogenous. Such industrial 3D lots must be converted into a 1D lot (in which the two width–height dimensions are negligible compared to the third processing dimension). In practice, this often means transferring the entire lot, without material losses, on a conveyor belt and collecting the samples during this process. This is admittedly a costly, time-consuming process, but it does guarantee representativity. With (very) large lots, there is always a desire to find a cheaper and logistically less demanding solution—always subject to the universal representativity demands.
And there is such a solution in the present case. The whole sampling problem could simply have been eliminated before the stockpile had been completed. Instead of sampling the 3D stocks, it is much easier to sample the 1D streams before they reach the terminal end of the conveyor belts used to build up the stockpile. The most efficient sampling always takes place while the lot is a moving stream, and this can easily be performed so as to guarantee representativity by using correctly designed, usually automatic, sample cutters at the relevant discharge point, and by applying material-dependent composite sampling. A much simpler, much cheaper and guaranteed TOS-compliant solution!
Lessons learned
In the present case, if the decision had been made based on the initial non-representative grab samples, the upgraded processing circuit would have been implemented at the wrong process location and the corresponding designed flowsheet would have been sub-optimal, if not useless. The incorrect sampling issues have instead been resolved with very little investment—an external audit and three automated samplers—which served both the expansion project as well as the daily production control and reconciliation obligations well.
The incorrect grab sampling practices showcased above would have resulted in unacceptable financial consequences in the form of a net loss of >2M €. This figure corresponds to the Total Investment Costs (TIC) of the initial by-product recovery circuit designed for the wrong process stream and was calculated based on simulation results through process modeling and simulation software based upon the biased data. The final TIC values are extremely sensitive to the circuit feed rate which determines the size (or number) of processing units necessary. In the present case, the feed rate of the originally selected process location was double that of the plant stream selected after the audit. This means that the original by-product recovery circuit was severely over-sized. Worse, 2/3 of the TIC of the original circuit was accounted for by gravity recovery equipment, which, however, is inefficient in the size range of the process stream selected, as revealed by the audit.
In the mining, and many other industries, business decisions and project evaluations are heavily dependent on representative sample collection along the entire value chain from exploration to closure.3,4 Sampling errors are invariably larger when samples are collected and, therefore, must be representative of several metals or properties simultaneously,5–7 such as was the case here where several new by-product metals were targeted. Only implementation of strict, TOS-compliant, sampling procedures at the earliest stage of a mining project will allow proper management of technical and economic risks by preparing for best possible business decisions through access to documented reliable data to be used to optimise a mine plan over the full Life of Mine (LOM) horizon—ultimately also a prerequisite for maximising the Net Present Value (NPV).
References
- R.J. Holmes, “Correct sampling and measurement—the foundation of accurate metallurgical accounting”, Chemometr. Intell. Lab. Syst. 74, 71–83 (2004). https://doi.org/10.1016/j.chemolab.2004.03.019
- D.A. Vogel and K.H. Esbensen, “WHAT are sampling errors—and WHAT can we do about them? Part 2: Sampling and weighing—different, but the same…”, Spectrosc. Europe 33(3), 28 (2021). https://doi.org/10.1255/sew.2021.a16
- F.F. Pitard, Theory of Sampling and Sampling Practice, 3rd Edn. CRC Press, Boca Raton, Florida (2019). https://doi.org/10.1201/9781351105934
- K.H. Esbensen, “TOS reflections: is there a third way? (to promote the Theory of Sampling)”, TOS Forum 10, 21 (2020). https://doi.org/10.1255/tosf.122
- Q. Dehaine, L.O. Filippov and J.J. Royer, “Comparing univariate and multivariate approaches for process variograms: A case study”, Chemometr. Intell. Lab. Syst. 152, 107–117 (2016). <https://doi.org/10.1016/j.chemolab.2016.01.016
- Q. Dehaine and L. Filippov, “A multivariate approach for process variograms”, TOS Forum 5, 169 (2015). https://doi.org/10.1255/tosf.76
- T.M.K. Le, Q. Dehaine, B. Musuku and N. Schreithofer, O. Dahl, “Sustainable water management in mineral processing by using multivariate variography to improve sampling procedures”, Miner. Eng. 172, 107136 (2021). https://doi.org/10.1016/j.mineng.2021.107136
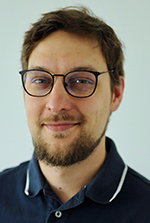
Quentin Dehaine
Quentin Dehaine is a Senior Researcher at the GTK with strong expertise in ore mineralogy, mineral processing and geometallurgy of battery metals (notably cobalt and lithium) and critical raw materials such as rare earths elements. He is particularly interested in the integration of geology, mining engineering, mineral processing and metallurgy through the application of geometallurgy. More generally, the focus of his research is to develop innovative integrated approaches to support mine value chain optimisation, responsible sourcing, reduce technical risk, maximise resource efficiency and minimise environmental impacts. Quentin holds an engineering degree from the Ecole Nationale Supérieur de Géologie and a PhD from the Université de Lorraine in France. Before joining GTK, he was a postdoc at the Camborne School of Mines in the UK. His expertise also covers waste valorisation, traceability, the Theory of Sampling and process modelling.
[email protected]